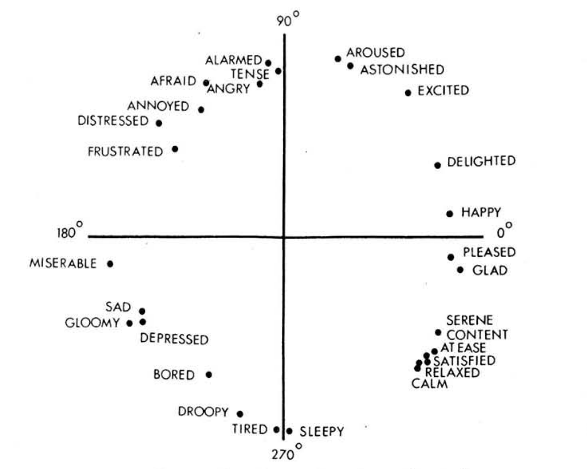
Abstract
Automatic annotation of music with emotion labels is a challenging task owing to the subjectivity of emotions associated with music. Much of this work focuses on creating a sizeable dataset with sufficient annotations that tackles the issue of subjectivity adequately. By combining pre-existing datasets with discrete and continuous emotion labels, a dataset with 79 hours worth of audio is obtained. All the audios are represented as points in the valence-arousal plane and are then clustered into four classes for the purpose of developing classifiers. CNNs which use mel spectrograms as inputs are the primary focus of this work. A maximum accuracy of 50.89% is obtained in the four-class classification task with accuracies in the individual tasks of valence and arousal classifications being 70.8% and 71.7% respectively.